Our latest LinkedIn poll on September 27th was focused on understanding the role and impact of artificial intelligence and machine learning (AI/ML) technology on the general identity and access management industry.
I attended the recent London versions of the Gartner Identity and Access Management summit and later Security & Risk Management in September and an anecdotal walk around the vendor booths (and there were about 50+ at each event) showed how AI/ML had become either a standard reference in the marketing narrative or was a road-map item to become a “competitive differentiator”.
The poll was a finger in the air check on the pulse as to where AI/ML might provide some assistance to the IAM world. The four areas chosen are all relatively mature (existing and startup vendors, budget allocation, stable use cases) and also potentially suffer from visibility and response issues. By this I mean either there is not enough instrumentation to identify issues, errors or anomalous behaviour or possibly the volume of activity associated with that area cannot be easily responded to by the human eye – or via existing manual process.
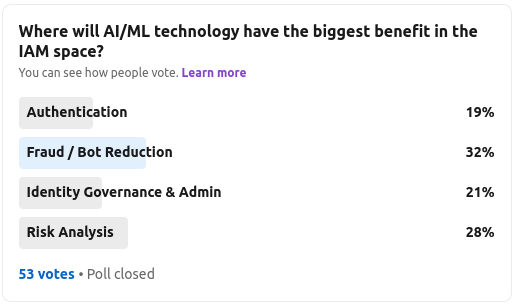
As always, the poll ran for a week and had 53 votes – mainly senior identity and security leaders, consultants and a few vendors representatives too.
The results I would say are not conclusive. Yes, there is a “winner” with fraud and bot reduction receiving 32% of the vote, but there was only 13% between 1st and 4th.
Let’s tackle each area in turn.
1 – Fraud / Bot Reduction
Fraud and bot activity is a huge issue for the financial services industry as well as the broader retail, eCommerce and consumer goods sectors. Gaming too has suffered from this in recent years, where the virtual-world style games often deliver opportunities for gamers to buy assets or upgrade players within a game for a price – allowing cyber criminals to hijack the intervening purchase and cash-out processes when assets are exchanged back for real currency. Fraud detection and management technology is well used within FSI and banking and identity is starting to add automated capabilities into this sector. Fraud detection often starts during identity registration and onboarding where proofing technology can help reduce bots and sythetic accounts as well as reduce automation style attacks. The use of AI/ML here seems sensible – lots of data, lots of money involved and the cost of getting the analysis wrong is quite large.
Examples Vendors: IDNow, OnFido, Veriff
2 – Risk Analysis
Clearly risk analysis is a more generic area and not specific to IAM, but risk assessment during many aspects of the identity life-cycle is becoming popular – with extra context being available and wanted by decision engines used during registration, access control decisioning, user to entitlements association, privilege escalation and so on. The output of this analysis is a risk “score”, which can sometimes be seen as a number as opposed to the traditional vendor demo showing “high”, “medium” or “low” risk. Both approaches are often shrouded in mystery with respect to how those quantitative or qualitative values are both created and more importantly interpreted. A couple of terms start to spring up here (some of which may be getting lifted from the IGA space) such as “identity analytics” or “identity intelligence”. The concept being there is some context being captured, analysed and presented to various decision points to help reduce risky situations. As we have lots of data to “analyse” seems sensible AI/ML may come to the rescue.
Example Vendors: Oort (“identity analytics), Gurucul (“identity & access analyics”), Illusive (“automated identity risk management”, Ping Identity (“API intelligence)
3 – Identity Governance & Administration
IGA (and access certification, identity audit and identity attestation before it) has been around a while. The early version 1 incarnation saw the the likes of Vaau (later Sun Role Manager and then Oracle Identity Analytics), Aveksa (later acquired by RSA) and Sailpoint (still going strong) tackle the problem of working out who should have access to what. Data extracts using CSV feeds where often the order of the day which leveraged ETL (extract, transform, load) tools to massage data so that correlation from different permissions systems could be obtained. These “identity lakes” would often contain feeds from systems as complex and exciting as SAP, RACF mainframe, obscure SQL permission tables and custom systems. Static rules would be run looking for separation of duties breaches or specific known “bad combinations” – such as orphan accounts, redundant accounts, shared accounts and so on. On top of that, line managers would be getting bombarded with emails to review the access of their staff. They didn’t know the answer and just ticked “allow” anyway. All in all IGA became a big data problem. Lots of data (too much?) and not many ways of efficiently working out who should have access to what (and why). Enter AI/ML. Surely structured learning could help here? Whilst only coming third in the poll, it seems a few vendors are pushing automation at this problem – namely as it speeds up the process, but also removes non-technical line managers from the task too, as they can be spending their time on things that are more productive. Like their jobs.
Example Vendors: Sailpoint (“intelligent identity governance), Saviynt (“smart identity”), Brainwave GRC (“identity analytics)
4 – Authentication
Authentication is becoming a large and broad market. Lots of specialist providers and many platform players and CSP (cloud service providers) now provide a range of options – from basic OTP through to push notifications and FIDO2/WebAuthn support – basically for “free”. However, authentication is still the main pinchpoint to both B2E and B2C application access and specialist capabilities are still needed. The “login page is the app” as seen by the end user – and it must deliver both usability and security requirements in one go. As authentication is starting to analyse more non-identity signals (think device, location, threat intelligence, breached credentials data and so on) it looks remarkably like another big-data problem. A problem which can’t be solved by people and static rules or signatures. What seems to be increasingly common is credential theft (aka stealing a username and password, or even a OTP in transit) so the question becomes not “is this credential correct”, but “is it being used by the correct identity”. That “correct identity” question is often asked by checking “has anything changed” since credential issuance time, credential use time and credential checking time. As such many authentication solutions (either via platforms or specialists) are adding AI/ML capabilities to validate good behaviour from bad, good devices from bad and so on.
Example Vendors: ForgeRock (“autonomous access”), Transmit Security (“advanced security” CIAM platform), OneSpan (“adaptive authentication), RSA (“ID plus”)
Summary
If “software is eating the world”, is perhaps AI/ML eating software? Or at least IAM software? If identity is really becoming a “data problem” due to the pervasive nature of workforce IAM and the breadth of coverage for consumer data integration, it seems perhaps AI/ML does have a significant role to play across many aspects of the identity life-cycle.
About the Author
Simon Moffatt is Founder & Analyst at The Cyber Hut. He is a published author with over 20 years experience within the cyber and identity and access management sectors. His most recent book, “Consumer Identity & Access Management: Design Fundamentals”, is available on Amazon. He has a Post Graduate Diploma in Information Security, is a Fellow of the Chartered Institute of Information Security and is a CISSP, CCSP, CEH and CISA. His 2022 research diary focuses upon “Next Generation Authorization Technology” and “Identity for The Hybrid Cloud”.